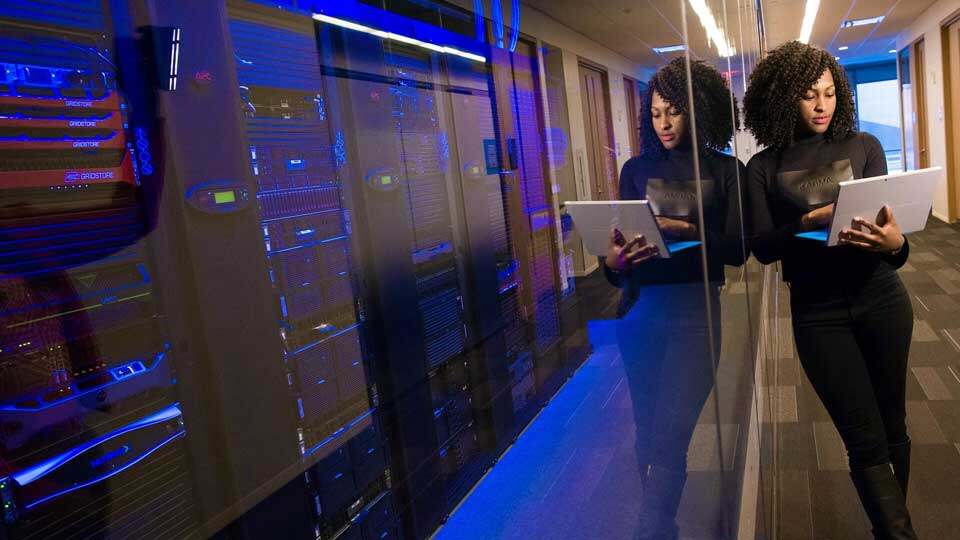
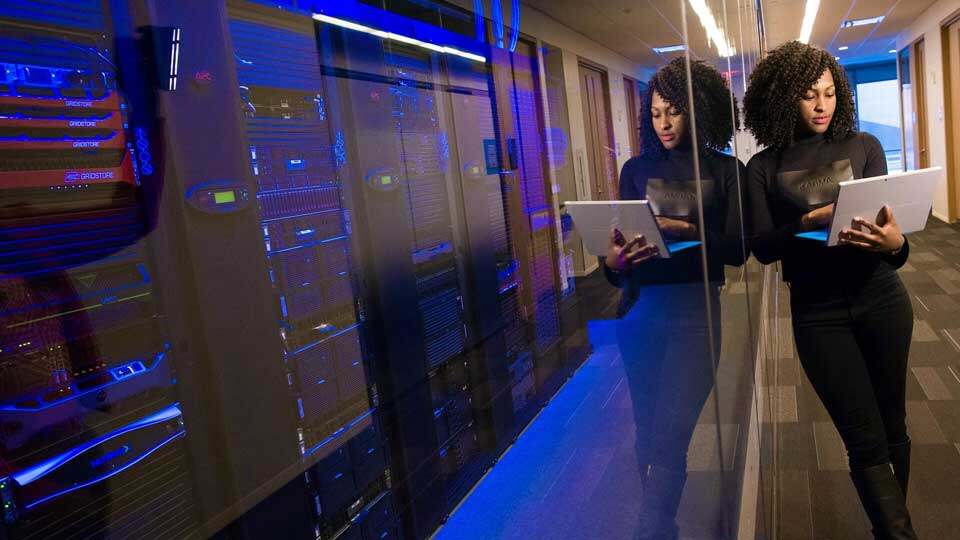
Under the hotly debated impacts of generative Artificial Intelligence (AI) lies the deeper problem of biased data teaching AI. Perhaps now more than ever, with growing numbers of people and organizations turning to tools such as ChatGPT to write their essays, legal briefs, or make critical decisions, the challenge of algorithmic bias and injustice built into AI systems is urgent.
While racial and gender discrimination are often cited among harms resulting from bias in AI, broader and interrelated areas of impact include job loss, privacy violations, healthcare discrimination, political polarization, and the spread of disinformation.
“AI is predominantly a white and male domain,” says Waterloo’s Dr. Carla Fehr, “and there is a pressing need for work on race, gender, disability, and social issues related to AI.”
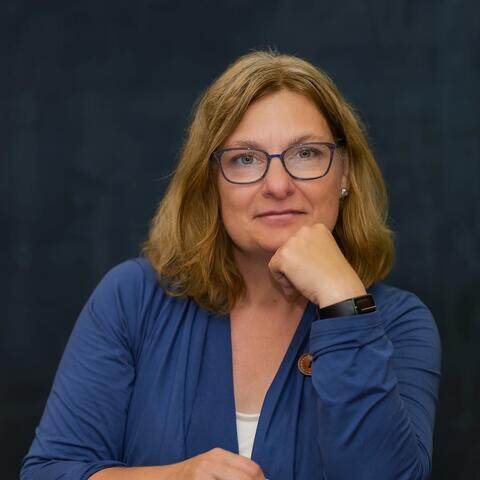
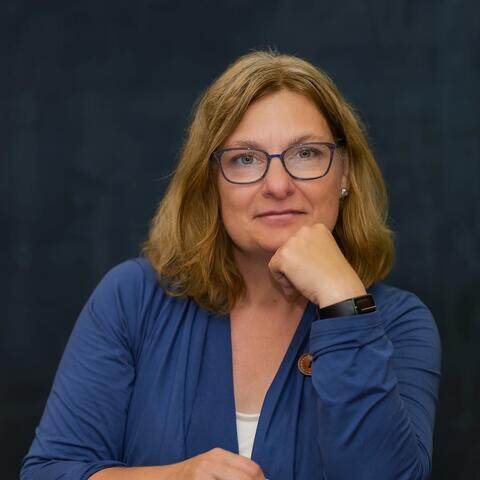
Fehr is an associate professor of philosophy and holds the Wolfe Chair in Scientific and Technological Literacy. In 2021 she convened the Feminism, Social Justice and AI workshop that invited scholars from her own field of feminist philosophy to collaborate with other experts including computer scientists, engineers, economists, policy makers, and industry experts. Their challenge was to explore ways to foster the responsible development and use of AI.
“We have such spectacular progress in terms of technological growth. And there’s a lot of really interesting work being done on social theory and ethics around AI. But for either of these fields to make a positive difference in the world, they need to talk to each other,” says Fehr.
Developing equity-informed algorithmic systems is not a simple task. But like other areas of injustice, it starts with identifying and understanding how harms are done within the existing system.
AI harms and helps
Years ago when she began studying social impacts of AI and reading books such as Safiya Noble’s Algorithmic Oppression and Cathy O’Neil’s Weapons of Math Destruction, Fehr was struck by how powerful and how dangerous machine learning algorithms can be. She gives the example of AI models used to predict recidivism – the likelihood of former offenders to reoffend. “Recidivism rates are significantly overpredicted for Black people and underpredicted for white people. Not only were these AI models reflecting really harmful social patterns that exist right now, but they were reinforcing and growing them.”
Of course, AI and machine learning does good too. “Some of these algorithms promise incredible contributions to human welfare,” writes Fehr in her introduction to an issue of Feminist Philosophy Quarterly dedicated to the Feminism, Social Justice and AI workshop papers. “For example, some AI-powered systems can detect very early stages of medical conditions, and others are being developed and used to combat human trafficking. Also, consider social media’s role in liberatory social movements such as Arab Spring, Black Lives Matter, and #MeToo.”
Diverse representation is key
Promoting diversity in STEM fields is an important area of Fehr’s scholarship that intersects with justice in AI. Put simply, diversity and justice lead to better science, she argues. And at the root of biased technological design is a lack of diversity among its developers.
She gives the example of Joy Buolamwini, a Black researcher at MIT who noticed facial recognition software that did a poor job detecting and frequently misgendered Black or dark-skinned women’s faces, so she pioneered critical work on addressing bias built into the technology. Fehr stresses that “people need to understand that racism is not just an individual person with a bad attitude, but that racism is built into many of our systems and institutions. And so improving education and employment equity are central to addressing these tech problems and making things better.”
Fehr is among a growing group of multi-disciplinary researchers at Waterloo advocating for responsible science and technology development, which includes initiatives such Tech for Good and Ethical by Design. Within the Faculty of Arts, scholars in Communication Arts, English, Philosophy, Sociology, the Stratford School, and more, engage in this work.
Toward feminist AI
But what do feminist approaches to rewriting algorithms look like? What would it take to create a feminist AI? “That’s so huge,” says Fehr.
Collectively, the participants in the Feminism, Social Justice and AI workshop demonstrated that there are important opportunities for feminist scholarship on the development of new algorithmic systems. For instance, their papers proposed steps such as: identifying specific barriers to debiasing algorithms; evaluating algorithms for their role in racial stratification; pushing tech companies to hire people with lived experience to curate autocomplete responses for racialized queries; and investigating the impacts of cultural code-switching in emerging AI technologies.
Fehr noted that in many of the papers written for the workshop a particular philosopher came up. “Iris Marion Young worked on structural injustice and developed the idea that the responsibility for an injustice is distributed among all the people who participate in it. And for understanding and addressing algorithmic bias and injustice in AI, I think that’s a really useful notion.”